Data is a production factor and plays an important role in the creation of products and services in all of today’s companies. Data can be used in various ways in production, such as in the improvement of production processes, the optimization of supply chains or the personalization of products and services. In Industrie 4.0 in particular, data is increasingly seen as an important production factor, as it forms the basis for many innovative technologies such as the Internet of Things, artificial intelligence and automated systems. These technologies can help to improve the efficiency, quality and flexibility of production processes and thus increase the competitiveness of companies.
However, it is important to note that data alone is not enough to create value for companies. To effectively use data as a production factor, companies must be able to collect, store, process and analyze it to gain insights and knowledge that help improve business processes. Therefore, it is of great importance for companies to improve their data strategy regarding the right data architecture and their technological capabilities in order to take full advantage of the use of data as a production factor.
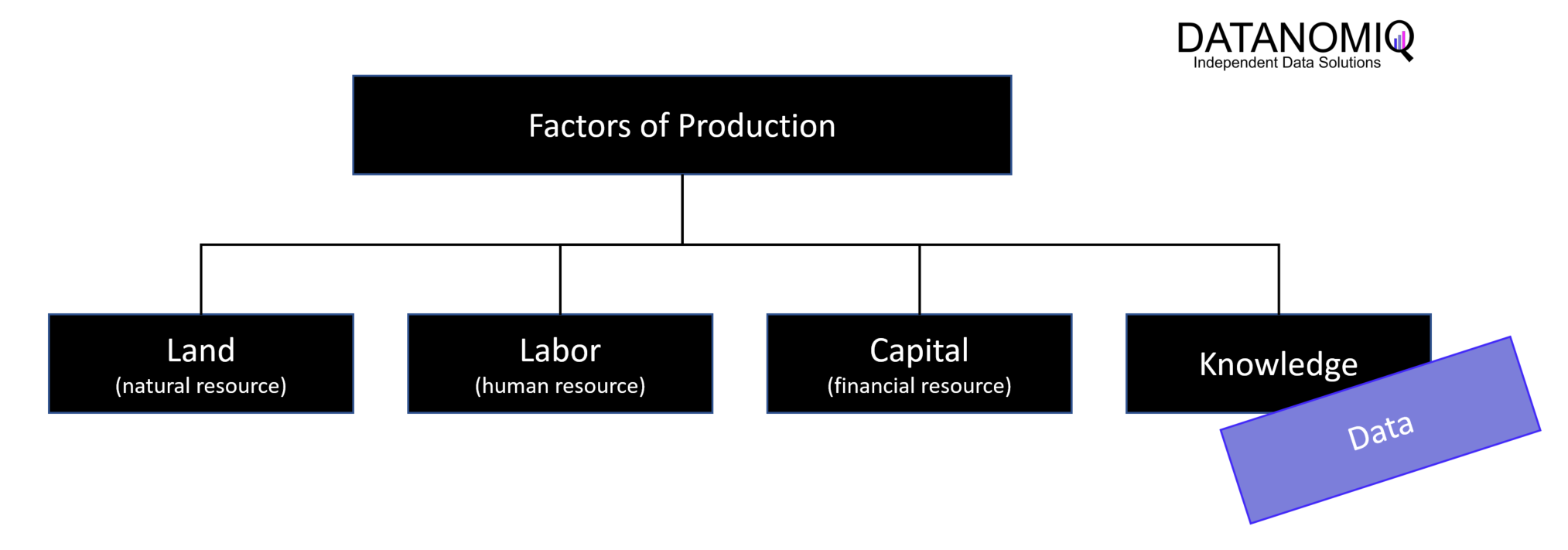
To use data properly as a production factor, it must be made available to all stakeholders effectively and efficiently.
Data availability, quality and transparency increase business valuation
Transparent processes improve collaboration between departments, qualitative data increases the efficiency of business processes and reduces the risk of errors. High data quality is also important for making informed decisions and avoiding potential legal problems. In any case, the availability of high-quality data and transparency about processes – including those for data preparation itself – increase business valuation, as investors and stakeholders can assess the company’s performance more accurately, more reliably and, above all, more quickly, and effective processes can help to increase profitability and manage the future.
Data readiness for Business Intelligence
Transparency about processes and the quality of data plays a central role for companies because it has a direct impact on the decision-making, efficiency and reliability of business processes. Business intelligence (BI) supports decision-making in various ways by facilitating access to relevant data and information. High data quality is critical in this regard, as organizations today are collecting more data than ever to make informed decisions. When data is incomplete, inaccurate or outdated, it can lead to poor decisions that can negatively impact the business. In addition, poor data quality can also cause legal problems if companies violate data protection laws, for example.
For business intelligence analyses and reports, data from various sources must be merged, cleansed, consolidated and prepared into target-oriented data models. Here, the Schema on Write principle prevails, which means that before data can be integrated into those data models, the necessary database tables must first be created, with the correct meta-descriptions about data types, storage sizes and prescribed links (relations). The effort to prepare data for BI is therefore already quite high before the actual use.
Data readiness for Process Mining
Process Mining, or Process Intelligence, is a subtype of business intelligence and enables organizations to monitor and measure processes more closely, which can lead to improvements in process quality and compliance with standards. Organizations can use it to ensure that their processes are effective and efficient and that they meet required quality standards. By analyzing process data, companies can identify bottlenecks, bottlenecks and inefficient workflows. These insights can be used to optimize and improve processes, which can lead to greater efficiency and productivity. Monitoring and error analysis for audit / compliance are also application purposes.
Similar to classic business intelligence, data must be available for process mining, qualitatively processed and summarized in data models (event logs).
Data readiness for Data Science and Artificial Intelligence
Companies can only benefit from Data Science and Artificial Intelligence (AI) if the data required for it is available and easily accessible to the Data Science teams and Machine Learning Engineers in the first place. Data Science also work with data according to the concept Schema on Read is an approach for processing data in Big Data environments. It refers to the fact that data does not have to be structured in a defined schema in advance, but only during the read operation directly after the data has been accessed. Unlike the schema-on-write method, where data must be converted to a fixed schema before the write operation, here data can simply be stored in a data lake or data pool in a straightforward manner, including very diverse structured and unstructured data and in a large mass. A data scientist can then make use of this data and does not have to call it in separately and at length.
Data Lakehouse as Solution
Thanks to the use of a data lakehouse, companies can achieve highly effective data availability while optimizing the efficiency of their data infrastructure and reducing costs in the process, since they do not have to manage multiple separate systems for data storage, data integration and data analysis. A data lakehouse derives from the concepts of the data warehouse and the data lake, providing a single platform that integrates all of these functions into one system.
Data Warehouse – The sorted filing cabinet
A Data Warehouse is a central database designed specifically for analysis and reporting. This centrality is underscored in its importance with the claim to be a single source of truth for a company or for a specific organization in terms of data and information.
It is a special type of database that collects, integrates, and cleanses data from multiple sources to provide a comprehensive and consistent view of the enterprise. A data warehouse differs from traditional databases in its ability to combine data from different sources and store it in a common structure. Data can come from internal and external sources such as ERP systems, CRM systems, log files and other sources.
Another feature of data warehouses is that they are typically used for decision support and reporting. The data in the data warehouse is prepared for analysis and reporting and transformed into meaningful information and reports.
Schema on Write (formatting on write) is an approach to processing data in a database or data warehouse. It refers to the fact that data must be structured in a specific schema or format when it is entered into the system. Data is not only cleaned and consolidated in a data warehouse, but also transformed into data models that are friendly for reporting, such as the star schema. The disadvantage derived from this is that data warehouses can be expensive and time-consuming, as data must be carefully cleansed and integrated to ensure that it is consistent and meaningful for the consuming applications (including BI tools for reporting).
Data Lake – The All Storage
In the Data Lake, the opposite happens compared to data warehouses, because in them data is stored in its original, non-structured form. A data lake is a scalable data platform on which structured and unstructured data can be stored, managed and analyzed. Unlike traditional databases, which are usually limited to a specific schema, a data lake is more flexible and can store large amounts of data of different types and sources.
Data Lakes are rarely used stand-alone, but are part of a data strategy that also provides for a data warehouse that is at least partially approached from the Data Lake. The data lake complements the overall data architecture with archives of data histories (e.g., for training AI), because with the schema on read approach (formatting on read), data is stored in its original, non-structured form in a data lake. Data can therefore be easily stored in the lake without much organization. This data can be in various formats such as text, JSON, CSV or other formats. A schema is applied to the data only when it is read from the data lake to load it into an analytic engine or other processing system.
This contrasts with Schema on Write, which, as it applies to the data warehouse, does not require data to be converted to a fixed schema before it is written.
Schema on Read offers more flexibility, especially for a Data Scientist, as it allows easier integration of different data sources and formats. It is particularly beneficial in environments with large and heterogeneous data sets, as it enables fast processing and analysis of data in different formats. However, a potential drawback of Schema on Read is that consistent data quality is hard to ensure due to the lack of uniform data structures and formats. Thorough data cleansing and transformation is therefore then necessary at the latest immediately before analysis in order to prepare data for meaningful analysis.
Data Lakehouse – The State of the Art All-Rounder
A Data Lakehouse is a new concept for data processing that represents a process-integrative combination of data warehouse and data lake. It is an approach that combines the flexibility and scalability of a data lake with the structuring and integration of data in a data warehouse.
A data lakehouse stores data in its native format, similar to a data lake. Data is stored in raw form and then transformed into a tabular form using structured metadata. However, unlike a data lake, data identified as particularly relevant is structurally processed, similar to a data warehouse. This means that a data lakehouse can store, search, process and link both structured and unstructured data.
An important advantage of a data lakehouse is that they can integrate and process both structured and unstructured data, which enables greater flexibility and scalability for versatile applications and brings a high degree of future-proofing. By combining data lake and data warehouse technologies, companies can access and process their data faster and more cost-effectively. This architecture is particularly suitable for data-intensive applications and Big Data analysis, while always being the central data source for all analytical applications (BI, process mining, data science, AI) as well as for subsequent operational IT systems.
Accordingly, a data lakehouse enables companies to access and process data faster and more efficiently. This enables them to use their data more effectively as a production factor and more quickly gain insights that help improve business processes.
DATANOMIQ is the independent consulting and service partner for business intelligence, process mining and data science. We are opening up the diverse possibilities offered by big data and artificial intelligence in all areas of the value chain. We rely on the best minds and the most comprehensive method and technology portfolio for the use of data for business optimization.